Chapter 14 군집해의 평가 및 해석
군집분석 수행 시에는 분류분석과 달리 각 객체가 속하는 군집에 대하여 알려진 학습표본이 없기 때문에 어떤 군집해의 성능을 평가하기가 곤란하다. 각 객체가 이차원 또는 삼차원의 변수로 이루어진 경우에는 각 객체를 좌표축에 나타내어 도식화함으로써 어느 정도 군집해의 타당성을 정성적으로 평가가 가능할 것이나, 이보다 높은 차원의 경우에는 도식화가 불가능하다. 따라서 응용분야에 따라 전문가의 견해가 군집해의 평가에 필요할 수도 있다.
14.1 필요 R package 설치
본 장에서 필요한 R 패키지들은 아래와 같다.
package | version |
---|---|
tidyverse | 1.3.1 |
cluster | 2.1.2 |
flexclust | 1.4-0 |
clValid | 0.7 |
14.2 군집해의 평가
군집해의 정량적인 평가척도에 대한 연구는 지속적으로 이루어지고 있으며, 크게 외부평가지수(external index)와 내부평가지수(internal index)로 구분되고 있다.
14.2.1 외부평가지수
이미 잘 알려진 군집해가 있다고 가정할 때, 새로 제안된 군집해를 기존에 알려진 군집해와 비교하는 평가척도이다.
\(n\)개의 객체에 대하여 알려진 기준 군집해를 다음과 같다고 하자.
\[\begin{equation*} \mathbf{U} = \{ U_1, U_2, \cdots, U_r \} \end{equation*}\]
즉, 기준 군집해 \(\mathbf{U}\)는 \(r\)개의 군집으로 구성되며, \(k\)번째 군집을 \(U_k\)로 나타낸다.
유사하게, 비교 대상의 군집해를 다음과 같이 \(s\)개의 군집으로 구성된 \(\mathbf{V}\)로 나타내자.
\[\begin{equation*} \mathbf{V} = \{ V_1, V_2, \cdots, V_s \} \end{equation*}\]
14.2.1.1 기본 R 스크립트
다음의 두 군집해 간의 유사도를 랜드지수 및 수정랜드지수를 이용하여 표현할 수 있다.
\[\begin{eqnarray*} U &=& \{ \{1, 2, 3, 4\}, \{5, 6, 7\}, \{8, 9, 10\} \}\\ V &=& \{ \{1, 2, 5, 8\}, \{3, 6, 9\}, \{4, 7, 10\} \} \end{eqnarray*}\]
랜드지수 및 수정랜드지수는 flexclust
패키지의 randIndex
함수를 호출하여 계산할 수 있으며, 랜드지수를 계산할 때는 correct = FALSE
, 수정랜드지수를 계산할 때는 correct = TRUE
로 파라미터 값을 지정하여야 한다. 파라미터값을 지정하지 않을 때는 기본값으로 수정랜드지수를 계산한다.
<- c(1, 1, 1, 1, 2, 2, 2, 3, 3, 3)
sol_1 <- c(1, 1, 2, 3, 1, 2, 3, 1, 2, 3)
sol_2 map(c(FALSE, TRUE),
~ flexclust::randIndex(x = sol_1, y = sol_2, correct = .x))
## [[1]]
## RI
## 0.5111111
##
## [[2]]
## ARI
## -0.25
14.2.1.2 랜드지수
두 군집해 \(U, V\) 내 에서 각 객체가 속한 군집을 나타내기 위한membership 변수를 아래와 같이 정의하자.
\[\begin{equation*} u_{ik} = \begin{cases} 1 & \text{ if object $i$ belongs to cluster $U_k$, i.e. } i \in U_k \\ 0 & \text{ otherwise} \end{cases}, \, i = 1, \cdots, n, \, k = 1, \cdots, r \end{equation*}\]
\[\begin{equation*} v_{ik} = \begin{cases} 1 & \text{ if object $i$ belongs to cluster $V_k$, i.e. } i \in V_k \\ 0 & \text{ otherwise} \end{cases}, \, i = 1, \cdots, n, \, k = 1, \cdots, s \end{equation*}\]
랜드지수를 산출하기 위해, 우선 다음과 같은 네 가지 값을 정의한다.
\[\begin{eqnarray*} a &=& \sum_{i = 1}^{n - 1} \sum_{j = i + 1}^{n} \sum_{k = 1}^{r} \sum_{l = 1}^{s} u_{ik} u_{jk} v_{il} v_{jl}\\ b &=& \sum_{i = 1}^{n - 1} \sum_{j = i + 1}^{n} \sum_{k = 1}^{r} \sum_{l = 1}^{s} u_{ik} u_{jk} v_{il} (1 - v_{jl})\\ c &=& \sum_{i = 1}^{n - 1} \sum_{j = i + 1}^{n} \sum_{k = 1}^{r} \sum_{l = 1}^{s} u_{ik} (1 - u_{jk}) v_{il} v_{jl}\\ d &=& \sum_{i = 1}^{n - 1} \sum_{j = i + 1}^{n} \sum_{k = 1}^{r} \sum_{l = 1}^{s} u_{ik} (1 - u_{jk}) v_{il} (1 - v_{jl}) \end{eqnarray*}\]
이 때, Rand (1971) 가 제안한 랜드지수(Rand index)는 다음과 같이 정의된다.
\[\begin{equation} RI = \frac{a + d}{a + b + c + d} \tag{14.1} \end{equation}\]
여기서, \(a + b + c + d\)는 객체 쌍의 전체 수를 의미하므로 다음과 같다.
\[\begin{equation*} a + b + c + d = {n \choose 2} \end{equation*}\]
식 (14.1)는 0에서 1 사이의 값을 갖게 되며, 0에 가까울수록 두 군집해가 일치하지 않음을, 1에 가까울수록 두 군집해가 일치함을 나타낸다. 또한 랜드지수는 랜덤하게 작성한 두 군집해 간의 유사도의 기대값이 0보다 크다.
Hubert and Arabie (1985) 는 수정랜드지수(adjusted Rand index)를 아래와 같이 제안하였다.
\[\begin{equation} RI_{adj} = \frac{2 (ad - bc)}{(a + b)(b + d) + (a + c)(c + d)} \tag{14.2} \end{equation}\]
식 (14.2)는 랜덤한 군집해 간의 비교의 경우 0에 가까운 값을 갖는다.
아래 구현한 rand_index
함수는 임의의 두 군집해 u
와 v
에 대한 랜드지수 및 수정랜드지수를 계산하는 함수이다.
u
,v
: 서로 비교할 두 개의 군집해 벡터. 각 원소값은 각 객체가 속한 군집을 나타낸다.- 다음과 같은 두 개의 component를 지닌 list를 리턴한다.
ri
: 랜드지수adj_ri
: 수정랜드지수
<- function(u, v) {
rand_index if (!is_vector(u) || !is_vector(v)) {
stop("Input needs to be vector")
else if (length(u) != length(v)) {
} stop("Vectors u and v must have the same length.")
}
<- tibble(
U i = seq_along(u),
cluster = u
%>%
) inner_join(
rename(., j = i),
by = "cluster"
%>%
) select(-cluster) %>%
filter(i < j)
<- tibble(
V i = seq_along(v),
cluster = v
%>%
) inner_join(
rename(., j = i),
by = "cluster"
%>%
) select(-cluster) %>%
filter(i < j)
<- nrow(inner_join(U, V, by = c("i", "j")))
a <- nrow(anti_join(U, V, by = c("i", "j")))
b <- nrow(anti_join(V, U, by = c("i", "j")))
c <- choose(length(u), 2) - (a + b + c)
d
<- (a + d) / (a + b + c + d)
ri <- 2 * (a * d - b * c) /
adj_ri + b) * (b + d) + (a + c) * (c + d))
((a
return(list(ri = ri, adj_ri = adj_ri))
}
50개의 객체에 대해 랜덤하게 할당된 군집해(K = 3
) 두 개를 비교하여 랜드지수와 수정랜드지수를 구해보자.
<- function(n, r, s) {
random_rand_index <- sample.int(r, size = n, replace = TRUE)
u <- sample.int(s, size = n, replace = TRUE)
v rand_index(u, v)
}
set.seed(500)
rerun(200, random_rand_index(50, 3, 3)) %>%
bind_rows() %>%
gather(key = "metric", value = "value") %>%
ggplot(aes(x = value, fill = metric)) +
geom_histogram(binwidth = 0.05) +
scale_x_continuous(limits = c(-1, 1)) +
scale_fill_discrete(
name = "index",
breaks = c("ri", "adj_ri"),
labels = c("Rand Index", "adjusted Rand Index")
+
) labs(
title = "Distribution of index values from 200 random assignments",
x = "index value",
y = "frequency"
)
## Warning: Removed 4 rows containing missing values
## (geom_bar).
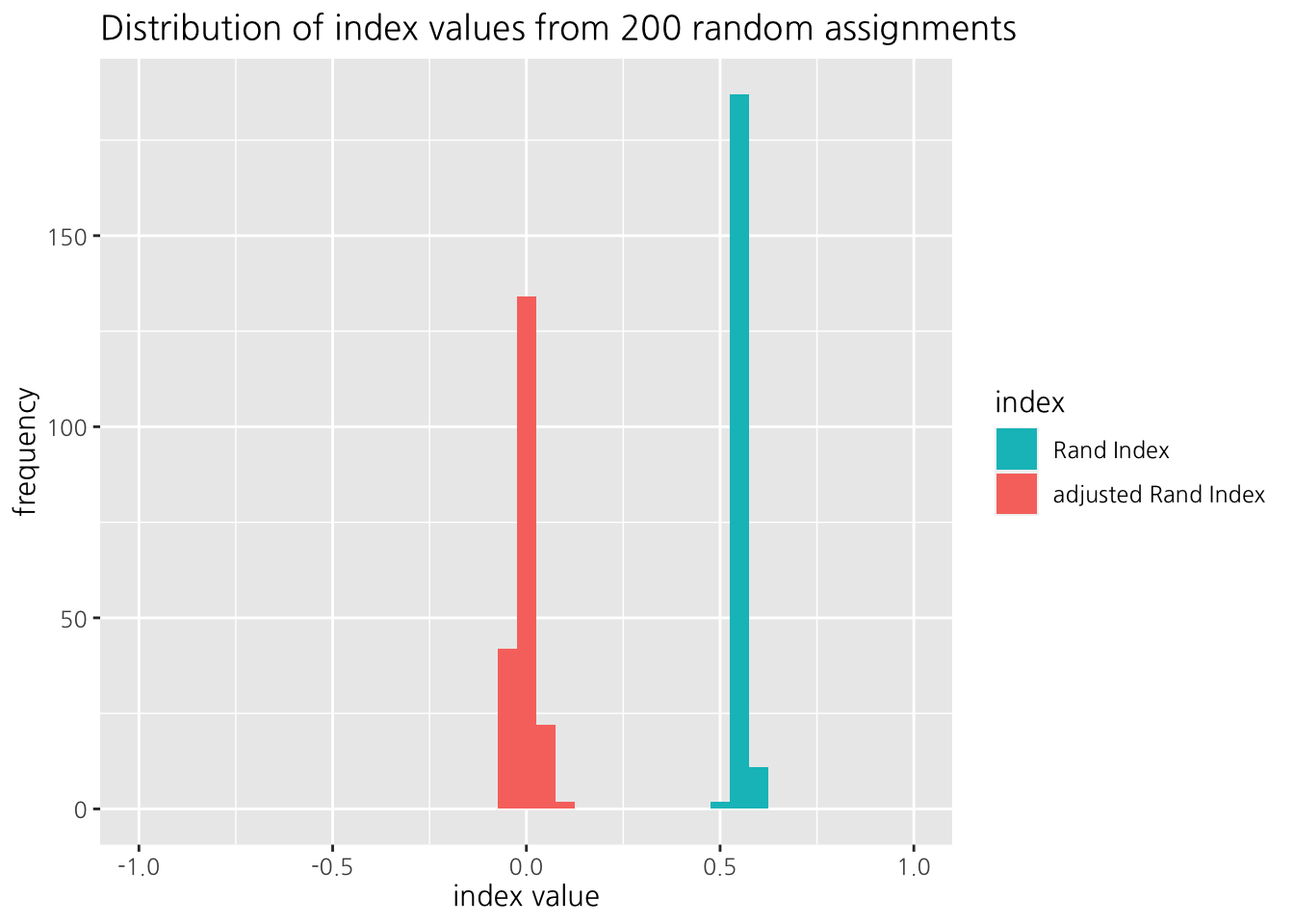
Figure 14.1: 랜덤한 군집해 간 비교: 랜드지수 및 수정랜드지수
Figure 14.1에 보이듯이, 랜덤한 군집해 간 비교에서 랜드지수는 0보다 큰 값을 나타내는 반면, 수정랜드지수는 0을 중심으로 분포되어 있다.
다음의 두 군집해에 대하여 랜드지수와 수정랜드지수를 구해보자.
\[\begin{eqnarray*} U &=& \{(1, 2, 3, 4), (5, 6, 7), (8, 9, 10) \}\\ V &=& \{(1, 2, 5, 8), (3, 6, 9), (4, 7, 10) \} \end{eqnarray*}\]
rand_index(
c(1, 1, 1, 1, 2, 2, 2, 3, 3, 3),
c(1, 1, 2, 3, 1, 2, 3, 1, 2, 3)
)
## $ri
## [1] 0.5111111
##
## $adj_ri
## [1] -0.25
14.2.2 내부평가지수
군집해에 대한 내부평가지수는 외부 정보의 도움 없이 입력 데이터만으로 군집해를 평가하는 척도로써, 주로 밀집성(compactness), 연결성(connectedness), 분리성(spatial separation) 등 세 가지 관점에서 평가한다.
우선 \(K\)개의 군집으로 이루어진 군집해 \(C\)가 다음과 같다고 하자.
\[\begin{equation*} C = \{ C_1, C_2, \cdots, C_K \} \end{equation*}\]
14.2.2.1 기본 R 스크립트
아래와 같이 두 개의 변수 \(x_1\) 및 \(x_2\)로 표현되는 객체 데이터에 대해 군집을 찾고자 한다.
<- tribble(
df ~id, ~x1, ~x2,
1, 4, 15,
2, 20, 13,
3, 3, 13,
4, 19, 4,
5, 17, 17,
6, 8, 11,
7, 19, 12,
8, 18, 6
)
%>%
df ::kable(
knitrbooktabs = TRUE,
align = c('r', 'r', 'r'),
col.names = c(
'객체번호',
'$x_1$', '$x_2$'
),caption = '군집 대상 데이터'
)
객체번호 | \(x_1\) | \(x_2\) |
---|---|---|
1 | 4 | 15 |
2 | 20 | 13 |
3 | 3 | 13 |
4 | 19 | 4 |
5 | 17 | 17 |
6 | 8 | 11 |
7 | 19 | 12 |
8 | 18 | 6 |
위 데이터에 대해 다음과 같은 두 개의 다른 군집해를 얻었다고 하자.
\[\begin{equation} \begin{split} U =& \{ \{1, 3, 6\}, \{2, 5, 7\}, \{4, 8\} \}\\ V =& \{ \{1, 3, 6\}, \{2, 4, 5, 7, 8\} \} \end{split} \tag{14.3} \end{equation}\]
<- c(1, 2, 1, 3, 2, 1, 2, 3)
sol_1 <- c(1, 2, 1, 2, 2, 1, 2, 2) sol_2
본 장에서는 4가지 내부 평가지수를 다룬다. 아래는 R 패키지들에 속한 각각의 함수를 실행하여 그 결과를 리스트 형태로 리턴하는 사용자 정의 함수 cluster_eval
를 구현한 것이다. 해당 함수에서 호출하는 R 패키지 함수들은 아래와 같다.
- Dunn index (Dunn 1973):
clValid::dunn
- CH index (Caliński and Harabasz 1974):
fpc::calinhara
- Connectivity (Handl and Knowles 2005):
clValid::connectivity
- Silhouettes (Rousseeuw 1987):
cluster::silhouette
각각의 평가지수에 대한 자세한 설명은 다음 장에서 하기로 한다.
아래 구현한 사용자 정의함수는 아래와 같은 입력 파라미터를 요구한다.
cluster
: 군집 해를 나타내는 길이 \(n\), 최대값 \(K\)의 정수형 벡터df
: 군집 데이터dist_method
: 거리 척도; Dunn, Connectivity, Silhouettes 지수 측정에 사용된다.nn
: 최근 객체 수 (>= 2); Connectivity 측정에 사용된다.
<- function(cluster, df, dist_method = "euclidean", nn = 2) {
cluster_eval <- clValid::dunn(
dunn_index Data = df,
clusters = cluster,
method = dist_method
)
<- fpc::calinhara(
ch_index x = df,
clustering = cluster
)
<- clValid::connectivity(
connectivity Data = df,
clusters = cluster,
neighbSize = nn
)
<- cluster::silhouette(
asw x = cluster,
dist = dist(df, method = dist_method)
"sil_width"] %>% mean()
)[,
return(list(
dunn_index = dunn_index,
ch_index = ch_index,
connectivity = connectivity,
asw = asw
))
}
map_dfr(list(sol_1, sol_2), cluster_eval, df = df[, -1], .id = "solution")
## # A tibble: 2 x 5
## solution dunn_index ch_index connectivity asw
## <chr> <dbl> <dbl> <dbl> <dbl>
## 1 1 1.08 26.5 1 0.651
## 2 2 0.822 15.4 0 0.586
14.2.2.2 대표 내부평가지수
Dunn (1973) 은 아래와 같은 지수를 제안하였다.
\[\begin{equation} DI = \frac{\min_{\mathbf{x} \in C_i, \, \mathbf{y} \in C_j \, 1 \leq i \neq j \leq K} d(\mathbf{x}, \mathbf{y})}{\max_{\mathbf{x} \in C_i, \mathbf{y} \in C_i, \, 1 \leq i \leq K} d(\mathbf{x}, \mathbf{y})} \tag{14.4} \end{equation}\]
여기서 분자는 군집 간의 분리성(클수록 분리성이 큼), 분모는 군집의 밀집성(작을수록 밀집성이 높음)을 반영하는 것이라 볼 수 있다. 따라서 분리성과 밀집성이 높을 때 식 (14.4)는 큰 값을 갖게 되며 해당 군집해가 상대적으로 좋게 평가된다.
군집해로부터 식 (14.4)를 계산하는 함수 dunn_index
를 아래와 같이 구현해보자. 입력 파라미터는 아래와 같다.
cluster
: 각 객체가 속한 군집을 나타내는 길이 \(n\)의 벡터df
: 객체 데이터를 나타내는 \(n\)행의 프레임dist_method
:base::dist
함수의method
파라미터값으로 사용할 거리 척도
<- function(cluster, df, dist_method = "euclidean") {
dunn_index # 객체 수
<- nrow(df)
n
# 각 객체의 군집해
<- tibble(
cluster_df id = 1:n,
cluster = cluster
)
# 각 객체 간 거리 데이터 프레임
# 위 군집해 데이터 프레임과 `inner_join` 을 통해
# 두 객체가 동일 군집에 속하는지 서로 다른 군집에 속하는 지 표현
<- dist(df, method = dist_method, upper = TRUE) %>%
dist_df ::tidy() %>%
broommutate_if(is.factor, ~ as.integer(as.character(.))) %>%
inner_join(
%>% rename(item1 = id, item1_cluster = cluster),
cluster_df by = "item1"
%>%
) inner_join(
%>% rename(item2 = id, item2_cluster = cluster),
cluster_df by = "item2"
)
# 서로 다른 군집에 속한 객체 쌍 중
# 가장 가까운 객체 간의 거리
<- dist_df %>%
numerator filter(item1_cluster != item2_cluster) %>%
top_n(-1, distance) %>%
slice(1) %>%
$distance
.
# 서로 같은 군집에 속한 객체 쌍 중
# 가장 먼 객체 간의 거리
<- dist_df %>%
denominator filter(item1_cluster == item2_cluster) %>%
top_n(1, distance) %>%
slice(1) %>%
$distance
.
<- numerator / denominator
res
return(res)
}
map_dbl(list(sol_1, sol_2), dunn_index, df = df[, -1])
## [1] 1.075291 0.822375
Caliński and Harabasz (1974) 는 다음과 같은 지수를 제안하였다.
\[\begin{equation} CH = \frac{\frac{1}{K - 1} \sum_{k = 1}^{K} n_k (\mathbf{c}_k - \mathbf{c})^\top (\mathbf{c}_k - \mathbf{c})}{\frac{1}{n - K} \sum_{k = 1}^{K} \sum_{i \in C_k} (\mathbf{x}_i - \mathbf{c}_k)^\top (\mathbf{x}_i - \mathbf{c}_k)} \tag{14.5} \end{equation}\]
여기에서 \(\mathbf{c}_k\)는 군집 \(k\)의 중심좌표(centroid), \(\mathbf{c}\)는 전체 객체들의 중심좌표, \(n_k\)는 군집 \(C_k\)내의 객체 수, \(n\)은 전체 객체 수를 나타낸다. 식 (14.5) 또한 분자는 분리성, 분모는 밀집성을 평가하며, 값이 클수록 좋은 군집해로 평가된다.
<- function(cluster, df) {
ch_index # 전체 데이터 중심
<- df %>% summarize_all(mean)
centroid
<- df %>%
cluster_df mutate(cluster = cluster) %>%
group_by(cluster) %>%
nest()
# 군집 중심
<- map_dfr(cluster_df$data, ~ summarize_all(., mean))
cluster_centroid_df
# 각 군집 중심과 전체 데이터 중심 간 제곱 유클리드 거리
<- flexclust::dist2(
centroid_dist
cluster_centroid_df,
centroid^2
)
# 각 군집 크기
<- map_dbl(cluster_df$data, nrow)
cluster_size
<- sum(centroid_dist * cluster_size) / (nrow(cluster_df) - 1)
numerator
<- map_dbl(
denominator $data,
cluster_df# 각 군집 내의 객체와 군집 중심 간 제곱 유클리드 거리의 합
~ flexclust::dist2(., summarize_all(., mean))^2 %>% sum()
%>%
) sum() %>%
`/`(nrow(df) - nrow(cluster_df))
<- numerator / denominator
res
return(res)
}
map_dbl(list(sol_1, sol_2), ch_index, df = df[, -1])
## [1] 26.45029 15.36218
Handl and Knowles (2005) 은 연결성을 반영한 아래와 같은 지수를 제시하고 있다.
\[\begin{equation} Conn = \sum_{i = 1}^{n} \sum_{j = 1}^{L} v_{i, nn_{i}(j)} \tag{14.6} \end{equation}\]
이 때, \(nn_{i}(j)\)는 객체 \(i\)의 \(j\)번째 최근 객체(nearest neighbor)를 나타내며, \(L\)은 연결성 척도 측정을 위한 사용자 지정 파라미터값이다. 또한 변수 \(v_{i, nn_i(j)}\)는 아래와 같이 정의된다.
\[\begin{equation*} v_{i, nn_i(j)} = \begin{cases} 0 & \text{ if } \exists C_k : i, nn_i(j) \in C_k \\ 1 / j & \text{ otherwise} \end{cases} \end{equation*}\]
즉, 식 (14.6)는 각 객체가 \(j (\leq L)\)번째 최근 객체와 다른 객체에 속하면 \(1 / j\)의 벌점을 부여하는 방식으로, 작은 값일수록 좋은 군집으로 평가될 수 있다.
<- function(cluster, df, dist_method = "euclidean", n_neighbor = 1) {
connectivity <- nrow(df)
n
<- tibble(
cluster_df id = 1:n,
cluster = cluster
)
# 객체간 거리
<- dist(df, method = dist_method, upper = TRUE) %>%
distance_df ::tidy() %>%
broommutate_if(is.factor, ~ as.integer(as.character(.)))
# 최근 객체
<- distance_df %>%
nn_df group_by(item1) %>%
mutate(nearest = rank(distance, ties.method = "random")) %>%
filter(nearest <= n_neighbor) %>%
ungroup() %>%
inner_join(
%>% rename(item1 = id, item1_cluster = cluster),
cluster_df by = "item1"
%>%
) inner_join(
%>% rename(item2 = id, item2_cluster = cluster),
cluster_df by = "item2"
)
# 연결성 계산
%>%
nn_df filter(item1_cluster != item2_cluster) %>%
mutate(v = 1 / nearest) %>%
$v %>%
.sum()
}
map_dbl(list(sol_1, sol_2), connectivity, df = df[, -1], n_neighbor = 1)
## [1] 0 0
map_dbl(list(sol_1, sol_2), connectivity, df = df[, -1], n_neighbor = 2)
## [1] 1 0
Rousseeuw (1987) 은 실루엣(silhouettes)이라는 내부평가지수를 제안하였다. 우선 다음과 같은 기호를 정의하자.
- \(a(i)\): 객체 \(i\)와 동일 군집에 속한 다른 객체들과의 평균 거리
- \(d(i, C_k)\): 객체 \(i\)와 다른 군집 \(C_k\)에 속한 모든 객체들과의 평균 거리, \(i \notin C_k\)
- \(b(i) = \min_{k: i \notin C_k} d(i, C_k)\)
객체의 군집 멤버쉽 변수 \(z_{ik}\)를
\[\begin{equation*} z_{ik} = \begin{cases} 1 & \text{ if } i \in C_k\\ 0 & \text{ otherwise } \end{cases} \end{equation*}\]
라 정의하면, 위 \(a(i)\)와 \(b(i)\)를 아래와 같이 수식화할 수 있다.
\[\begin{eqnarray*} a(i) &=& \sum_{k = 1}^{K} z_{ik} \frac{\sum_{j \neq i} z_{jk} d(\mathbf{x}_i, \mathbf{x}_j)}{\sum_{j \neq i} z_{jk}}\\ b(i) &=& \max_{k: z_{ik} \neq 1} \frac{\sum_{j \neq i} z_{jk} d(\mathbf{x}_i, \mathbf{x}_j)}{\sum_{j \neq i} z_{jk}} \end{eqnarray*}\]
이 때 객체 \(i\)에 대한 실루엣은 아래와 같이 정의된다.
\[\begin{equation} s(i) = \frac{b(i) - a(i)}{\max \{ a(i), b(i) \}} \tag{14.7} \end{equation}\]
식 (14.7)은 -1과 1 사이의 값을 갖는데, 1에 가까울수록 객체 \(i\)가 비슷한 객체들과 군집된 것으로, -1에 가까울수록 먼 객체들과 군집된 것으로 판단할 수 있다.
객체 \(i\)가 어떠한 다른 객체와도 같은 군집에 속하지 않는 경우가 발생할 수 있다 (\(i \in C_k, \, \left| C_k \right| = 1\)). 이 경우 \(a(i)\)가 정의되지 않으므로 \(s(i)\)값이 식 (14.7)에 의해서 정의되지 않는다. 이러한 경우에는 \(s(i) = 0\)이라고 실루엣을 정의한다.
\[\begin{equation} s(i) = \begin{cases} \frac{b(i) - a(i)}{\max \{ a(i), b(i) \}} & \text{ if $a(i)$ is defined}\\ 0 & \text{ otherwise } \end{cases} \tag{14.8} \end{equation}\]
이후 군집해의 평가지표로써 평균실루엣(overall average silhouette width; ASW)을 다음과 같이 정의하여 사용한다.
\[\begin{equation} ASW = \frac{1}{n} \sum_{i = 1}^{n} s(i) \tag{14.9} \end{equation}\]
<- function(cluster, df, dist_method = "euclidean") {
asw <- nrow(df)
n
<- tibble(
cluster_df id = 1:n,
cluster = cluster
)
<- dist(df, method = dist_method, upper = TRUE) %>%
dist_df ::tidy() %>%
broommutate_if(is.factor, ~ as.integer(as.character(.))) %>%
inner_join(
%>% rename(item1 = id, item1_cluster = cluster),
cluster_df by = "item1"
%>%
) inner_join(
%>% rename(item2 = id, item2_cluster = cluster),
cluster_df by = "item2"
)
<- dist_df %>%
dist_df group_by(item1, item1_cluster, item2_cluster) %>%
summarize(
avg_distance = mean(distance)
)
<- dist_df %>%
a filter(item1_cluster == item2_cluster) %>%
$avg_distance
.
<- dist_df %>%
b filter(item1_cluster != item2_cluster) %>%
top_n(-1, avg_distance) %>%
slice(1) %>%
$avg_distance
.
<- map2_dbl(a, b, ~ (.y - .x) / max(.x, .y))
s
mean(s)
}
map_dbl(list(sol_1, sol_2), asw, df = df[, -1], dist_method = "euclidean")
## `summarise()` has grouped output by 'item1', 'item1_cluster'. You can override using the `.groups` argument.
## `summarise()` has grouped output by 'item1', 'item1_cluster'. You can override using the `.groups` argument.
## [1] 0.6507364 0.5864226
14.3 군집해의 해석
군집분석의 주목적을 달성하기 위해서는 군집해를 얻은 후 이에 대한 해석이 가능하여야 할 것이다. 즉, 각 군집의 특성을 파악할 수 있어야 실제 문제에 적용할 수 있을 것이다. 이를 위해서는 특정 응용분야의 전문가 지식을 요하는 경우가 많다. 그러나 첫 출발은 각 군집의 중심좌표, 즉 군집 별 변수별 평균치를 산출하는 것이다. 대부분의 경우 변수별 평균치로 군집들을 비교함으로써 군집의 특성을 파악할 수 있다. 다변량을 처리할 수 있는 그래프 역시 도움이 되며, 특히 다변량인 경우 요인분석(factor analysis)를 활용하기도 한다. 최종적으로 각 군집에 대한 특성이 파악되면 명명(naming)하는 것이 추천된다.